Preliminary Course
Applied Mathematics
Probabilities. Discrete random Variables
Discrete Random Variable
-
Formally, a random variable X is a measurable function from a probability space (Ω, F, P)
to a measurable set (E,ε).
More intuitively, let us consider chance experiments (or events) with a finite number or countable infinite number of possible outcomes.
That is, the possible values might be listed, although the list might be infinite. The sample space Ω is the set of possible outcomes of the experiment.
For example, we roll a dice and the possible outcomes are 1, 2, 3, 4, 5, and 6 corresponding to the side that turns up.
A discrete random variable can then simply be considered as a function whose values correspond to the possible outcomes of a given chance experiment, possibly after some transformation is applied.
For example, the outcome of the roll of one dice is a random variable X.
And the sum of the outcomes of four independent dice rolls, Y = X
A famous historical example
-
A dice game played an important historical role at the origins of the probability theory.
Some famous letters between Pascal and Fermat were instigated by a request for help from a French nobleman and gambler, Chevalier de Méré.
It is said that de Méré had been betting that, in four rolls of a die, at least one six would turn up.
He was winning consistently and, to get more people to play, he changed the game to bet that, with a simple homothetic reasoning, in 24 rolls of two dice, a pair of sixes would turn up.
However, the exact probability value is a bit more complicated than that: it is said that de Méré lost with 24 and, disappointed, considered that mathematics was wrong.
In fact:
- 1 - (5/6)4 = 0.5177... > 0.5
1 - (35/36)24 = 0.4914... < 0.5
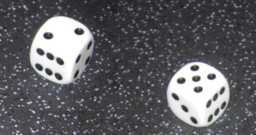
Dices (Photo M. Jaeger, CIRAD)
Discrete Random Function
-
The probability mass function p of a discrete random variable X satisfies the following properties:
1. The probability that the random variable X can take a specific value j is p(j). That is:
- ∀ j ∈ Ω
P[X=j] = p(j) = pj ≥ 0
2. The sum of p(j) over all possible values of j is 1, that is
- Σj pj = 1
where j represents all possible values that X can take.
A consequence is that 0 ≤ pj ≤ 1