Applications
Measurements
Fitting functional parameters
Overview
-
Since plant growth can be modelled in the form of a dynamic system,
classic methods of parameter estimation can be used, based on
maximum likelihood criteria and Newtonian methods of optimization.
The model outputs from which this identification can be achieved are the organ masses, as they can be easily measured on real plants and as they result from plant functioning and thus keep track of the whole history of source-sink balances.
If we consider a monospecies population, several plants at different ages can be used simultaneously to form the observation vector.
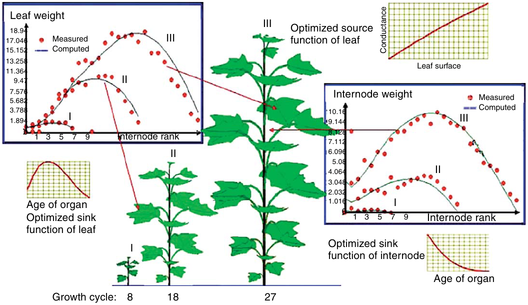
Fitting parameters from several growth stages (Zhang Zhighan, LIAMA/CAU 2008)
- Several growth stages of the same plant are fitted simultaneously at organ biomass level
with an optimized common set of parameters that ensure biomass production and biomass partitioning.
The organs of different types are fitted together because they share the same plant demand and production.
Plant growth dynamics may be controlled by a small set of constant parameters.
Complications can be induced if the population has strong intraspecific genetic variability and environmental variability.
The amount of data collected is a compromise between the statistical accuracy of estimation and the heaviness of the measurements.
In most cases, the simplifications used in the physiological model are justified by the comparison of the model with real plants, since a very small number of model parameters are sufficient to predict a large number of data.
Even though all the complex phenomena underlying plant growth and development are not accounted for, the prediction ability of such models remains quite good.
The reason is that the simple theoretical plant given by the model is such that its architectural trajectory is very close to that of the real complex plant.

Simulations of 3-D plant architectures including growth and development
(using versions of the GreenLab model: developed at INRIA, ECP, LIAMA, CIRAD)
(a) Arabidopsis plant, LEPSE (Digiplante software: ECP)
(b) Beetroot plant, Institut Tecnique de la Betterave (Digiplante software)
(c) Wheat plant, Wageningen University (GreenScilab software: LIAMA)
(d) Maize plant, Chinese Agricultural University (software CAU)
(e) Sunflower plant: INRA/LEPSE (Digiplante software)
(f) Chrysanthemum plant,Wageningen University (GreenScilab software)
(g) Pine tree, Chinese Academy of Forestry (Digiplante software)
(h) Coffee tree, CIRAD (Digiplante software)
(i) Cucumber plant, CAU (Digiplante software)
(j) Tomato plant, CAU (CornerFit software: LIAMA)
Compared to structural simulations, the representations of the above simulations are more accurate, because the sizes of organs depend on biomass production and biomass partitioning and do not directly result from empirical data sets.